Racialization in Testosterone Research
This project uses a mix of qualitative and computational methods to examine how population terms are used in testosterone research and the impact that this has on racial disparities in biomedical and behavioral research.
sts race gender networks qualitative ethicsKramer, B.L. (2020) “The Molecularization of Race in Testosterone Research.” BioSocieties. GitHub Repo.
While feminist science studies scholars have documented the misleading and dangerous implica-tions of reducing testosterone to a “sex hormone,” few studies have explored how testosterone is used to racialize populations in and beyond scientific research. In this article, I conduct a content analysis of 149 studies that evaluate population differences in testosterone. Despite widespread claims that testosterone varies between racial groups, my analysis of this literature provides scant evidence to support these proclamations, undermining the notion that testosterone contributes to racial differences in biomedical and biosocial outcomes. To supplement these findings, I use network analysis to visualize study outcomes as a citation network and trace racial differences testing in testosterone research from early 20th-century eugenics research to the contemporary “gold-standards” used by scientists today. Lastly, I propose three theoretical mechanisms – ambiguity, absence and data recycling – to help explain how the racialization of populations is perpetuated in this context. Together, these mechanisms provide a basis for STS scholars to better understand how researchers enact population differences across different scientific contexts.
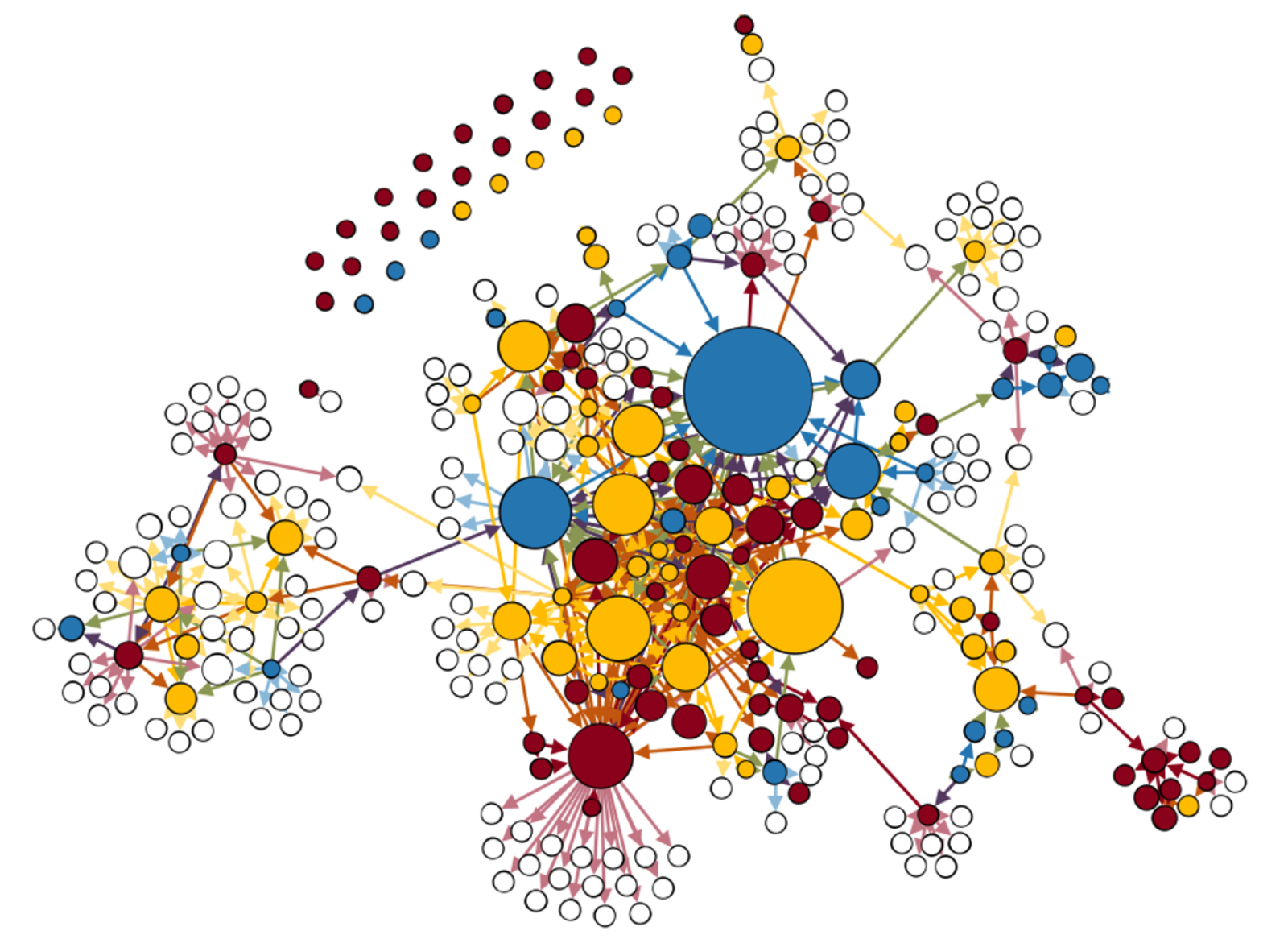
This is a network depicting 149 studies that test population differences in testosterone from 1966-2017. The nodes represent publications while the ties represent claims about population differences in testosterone. The size of the node signifies the number of times each study is cited within the network and colors of the nodes align with the outcomes of studies - red meaning no differences in testosterone, yellow meaning mixed results, and blue meaning clear differences in testosterone between the populations being tested. My analysis reveals that the majority of existing fails to find difference in testosterone between racialized populations, despite the fact that these claims are widespread throughout the literature.
Kramer, B.L. “Race as Relational Discourse: Using Network Analysis to Study Racial Claims in Scientific Research.” In Progress. GitHub Repo.
Science & technology studies scholars have investigated on how race has been molecularized within scientific studies. And while existing research mainly leans on qualitative strategies such as ethnographies, interviews, and rigorous historical analysis, very little work to date has used social network analysis to study how racial difference is constructed in scientific literature. In this study, I use discourse analysis to first codify racialized claims in a sample of studies that test population differences in the hormone testosterone. In turn, I construct a citation network where these racialized claims link to other publications in the network - creating a relational field where racialized discourses can be studied visually and statistically over time. Although little evidence actually exists to support researcher’s claims of population differences in testosterone, this paper goes beyond this lack of evidence by deploying an inferential strategy known as exponential random graph modeling (i.e. logistic regression for network data) to test which factors about these publications actually drive tie formation (i.e. racialized claims) within the context of this discursive field. My results show that racialized claims are shaped by a number of paper-level attributes such as scientific domain, the gender and location of the populations being studied, and the location of the researchers contributing to the manuscript in addition to various endogenous factors that control for the structure of the network. By synthesizing existing theories of the fields of bibliometrics and race & ethnicity studies, I not only provide a methodology that allows scholars to test racialization in science as a statistical phenomenon, but also provide STS scholars a framework to integrate observations from past and ongoing qualitative research that can formally test theories about the construction of racial difference in scientific research.